Effective Board Oversight as AI Evolves
January 16, 2025
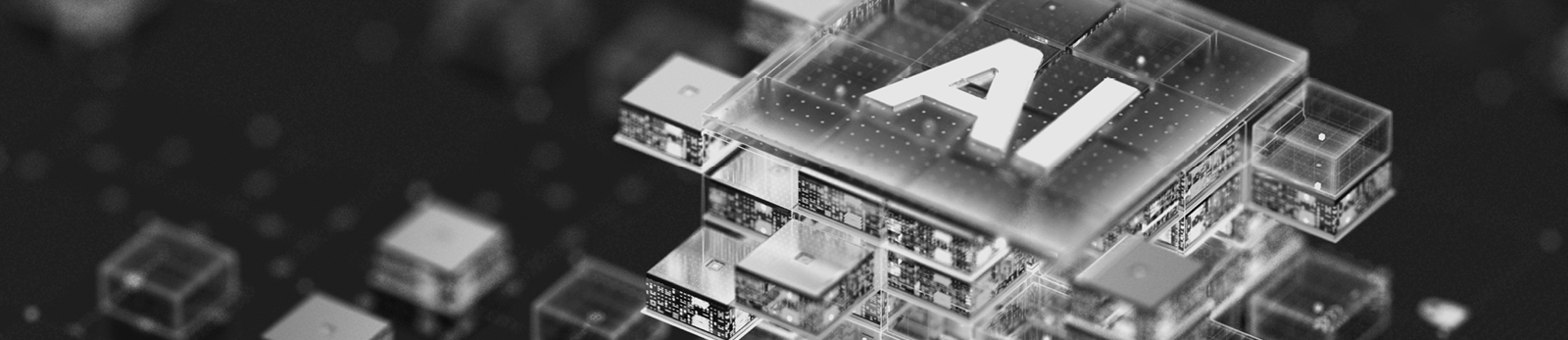
Deployment of generative AI expanded rapidly across many industries in 2024, leading to broadly increased productivity, return on investment and other benefits.
At the same time, AI was also a focus for lawmakers, regulators and courts. There are currently 27 active generative AI litigation cases in the U.S., nearly all of which involve copyright claims. Numerous state legislatures have mulled AI regulation, and Colorado became the first and only state thus far to pass a law creating a broad set of obligations for certain developers and deployers of AI.
Though Congress has yet to seriously engage with AI legislation, the SEC and the FTC have been using existing laws to bring AI-related enforcement actions. Numerous other federal agencies have hinted at potential regulation of AI,[1] but the future of U.S. AI regulation is uncertain given the new administration and upcoming turnover in regulatory leadership. Meanwhile, the EU’s Regulation No. 1689 (the EU AI Act) entered into force after three years of legislative debate.[2]
As the SEC steps up its enforcement against “AI washing”—making false or misleading claims about the use of AI in one’s business—it remains critical for boards of directors to manage AI risks with an in-depth understanding of how AI is used in their businesses.[3]
The Open Questions in U.S. Generative AI Copyright Litigation
Overview of AI Copyright Litigation
Whether training AI on copyrighted works constitutes fair use is a central issue in all 27 of the active generative AI cases. Under section 107 of the U.S. Copyright Act, the fair use of a copyrighted work, including for such purposes as criticism, commentary, news reporting, research or scholarship, is not copyright infringement. Courts consider four factors in determining whether a particular use is fair: (1) the purpose and character of the use, (2) the nature of the copyrighted work, (3) the amount and substantiality of the portion used in relation to the copyrighted work as a whole and (4) the effect of the use upon the potential market for or value of the copyrighted work. The primary inquiry is whether the challenged use is transformative, serving a different purpose or function from the original, or merely usurps the market for the original by reproducing it.
The first court to reach a decision on fair use in the context of an AI-augmented platform will likely be Thomson Reuters Enterprise Center GmbH v. ROSS Intelligence Inc.[4] In May 2020, Thomson Reuters sued ROSS Intelligence for allegedly copying headnotes from Westlaw, Thomson Reuter’s legal research platform, to train its AI-based legal research platform. ROSS argues that it made fair use of the Westlaw material, while Thomson Reuters argues that ROSS used content from Westlaw to build a directly competing platform without its authorization. In December 2024, the court held a lengthy hearing on the parties’ competing fair use positions at summary judgment, but no decision has yet been issued. If fair use cannot be resolved at summary judgment and the case proceeds to trial in May 2025, it will be the first AI copyright case to do so. Although the technology at issue in this case involves more traditional machine learning algorithms, it is being closely watched by litigants in the generative AI cases.
The first generative AI class action to reach summary judgment on fair use will almost certainly be Kadrey et al. v. Meta Platforms, Inc.[5] In this putative class action, a group of authors allege that Meta trained its Llama large language models on the text of their books without authorization. There are no allegations that the Llama models have been used to generate content resembling plaintiffs’ books. Rather, the theory is strictly that using the books for training constitutes copyright infringement. Summary judgment on fair use is set to be heard in May 2025. Decisions in Kadrey have already proven influential in narrowing the claims asserted in AI litigation across the country,[6] and the court’s ruling on fair use may do the same.
A fair use decision is also expected soon in Concord Music Group et al. v. Anthropic PBC.[7] There, dozens of music publishers allege that Anthropic infringed their rights by using copyrighted song lyrics to train Claude, Anthropic’s large language model.[8] To date, this case is the only generative AI copyright case in which the plaintiffs have sought preliminary injunctive relief. In opposition to the motion, Anthropic asserts that Concord cannot establish irreparable harm, that fair use makes success on the merits unlikely and that a decision on fair use before discovery would be premature. The motion for preliminary injunction has been fully briefed and argued, and a decision is expected in early 2025.
In addition to cases focused on AI training, a number of cases assert direct (as opposed to class) actions based on allegedly infringing outputs. In The New York Times Co. v. Microsoft Corp. et al.,[9] for example, the New York Times sued OpenAI, alleging that ChatGPT can replicate the exact content of articles otherwise available only behind a paywall. OpenAI claims the plaintiffs engineered the chat prompts to obtain the allegedly infringing outputs in a manner that does not emulate real-world use.
Andersen, et al. v. Stability AI Ltd., et al,[10] a putative class action by a number of visual artists against several leading generative AI image and video developers such as Midjourney, presents a question of first impression: whether artists can claim a generative AI model infringes their trade dress. The artists claim that Midjourney should be held vicariously liable for trade dress infringement when Midjourney tools are used to create outputs that plaintiffs allege replicate their art styles. This novel claim tests the boundaries of trademark protection for “style,” to which no copyright protection attaches, and will proceed to discovery and summary judgment along with the training-based copyright claim.
Regulatory Guidance on Copyright & AI
The courts will have final word on the fair use question, but the U.S. Copyright Office has promised further guidance on a number of other intellectual property issues, including the copyrightability of works created using generative AI, fair use in training AI, licensing considerations and allocation of potential liability between AI developers and users. The first installment of this guidance—on the use of digital technology to replicate an individual’s voice or appearance—was released in July 2024.[11]
Legislating AI in 2024
U.S. State Legislative Trends
While courts continue to grapple with novel AI copyright issues, state lawmakers are turning their attention to AI in a number of other contexts. For example, California, New York and other states have considered bills addressing discrimination by automated decision-making tools (ADMT). New York’s state legislature has proposed several bills that would limit use of ADMT by state agencies and an “AI Bill of Rights” that would provide New York residents with certain protections against use of ADMT without human intervention.
Numerous state legislatures also considered bills pertaining to the use of AI in employment contexts. These bills focused on areas such as providing notice to employees or potential employees of AI usage, limiting AI employee monitoring and identifying bias in employment decision tools. In addition, many state lawmakers focused on consumer protection and transparency—specifically, making consumers aware they are interacting with AI. For example, Utah passed its AI Policy Act,[12] which requires entities to disclose that a consumer is interacting with generative AI upon consumer request, or without request if AI is used by the entity in certain regulated occupations. California Senate Bill No. 942, effective January 1, 2026, similarly aims to facilitate consumer awareness of AI usage by requiring persons who create generative AI systems with over one million monthly visitors or users (and that are publicly accessible within California) to make an AI detection tool available at no cost. Finally, California AB 2013, effective January 1, 2026, requires developers of generative AI to provide, on their website, a high-level summary of the datasets used in developing their system, including whether the system uses synthetic data generation.
The Colorado AI Act
Colorado Senate Bill 24-205 Concerning Consumer Protections in Interactions with Artificial Intelligence Systems (the Colorado AI Act) was passed on May 17, 2024 and became the first law in the U.S to create a broad set of obligations for developers and deployers of certain AI systems.[13]
The Colorado AI Act, effective February 1, 2026, requires both developers and deployers of high risk AI systems[14] to use reasonable care to avoid algorithmic discrimination in their AI systems. To create a rebuttable presumption of reasonable care, developers and deployers must take certain actions such as publishing information about their AI systems and instituting a human-review appeals process for adverse consequential decisions. While there is no private right of action associated with the Colorado AI Act, it is enforceable by the Colorado Attorney General and can carry penalties of up to $20,000 per violation. The Colorado AI Act applies so long as the developer or deployer does business in Colorado.
Federal AI Regulation
In contrast, 2024 did not bring significant new proposals for AI regulation at the federal level. One proposal in the House of Representatives in the last Congress, would require AI developers to disclose whether and which copyrighted works were used to train models.[15] The bill would need to be reproposed in the current Congress to move forward. Additionally, the National Institute of Standards and Technology has continued to provide non-binding guidance for managing AI risks.[16] And, as discussed further herein, some federal agencies are using existing laws and regulations to promote responsible use and innovation of AI.
The EU AI Act
Compared to the U.S., the EU has been relatively proactive in regulating AI with its passage of the EU AI Act. The EU AI Act adopts a sliding scale of regulatory requirements depending on the level of risk posed by the AI system. Most AI systems currently used in the EU (e.g., spam filters or AI-enabled video games) will likely be categorized as minimal risk and will not be covered by binding rules. The EU AI Act imposes stringent obligations with respect to AI systems classified as high risk[17] and outright prohibits a narrow set of AI system applications, including biometric categorization systems based on sensitive characteristics.
The EU AI Act also imposes specific obligations on providers of general purpose AI (GPAI) models, such as maintaining technical documentation of the model, providing detailed information to downstream providers, implementing a policy to comply with EU law on copyright and related rights and publishing a summary of the training data.[18] The EU AI Act further imposes specific transparency requirements on providers of certain consumer-facing AI systems (e.g., chatbots), such as making users aware they are interacting with AI and labelling AI-generated content as such.
The EU AI Act has a broad jurisdictional hook, applying to any company whose AI is placed on the market or put into service in the EU, or whose AI output is used in the EU. The EU AI Act will apply directly across all EU member states, though most its provisions take effect only after a two-year transitional period. Failure to comply with its strictest provisions—those relating to prohibited AI systems—may result in fines of up to €35 million or 7% of group global annual turnover (whichever is higher). Non-compliance with most other provisions may result in fines of up to €15 million or 3% of group global annual turnover (whichever is higher).
AI Enforcement Actions in the U.S.
The SEC has recently ramped up its enforcement of AI washing claims. In March 2024, the SEC settled charges against two investment advisers, Delphia and Global Predictions, for false and misleading statements about their AI capabilities in violation of the Advisors Act.[19] According to the SEC, Delphia falsely claimed to use machine learning in its investment selections, and Global Predictions falsely claimed to be the “first regulated AI financial adviser” and exaggerated its use of “expert AI-driven forecasts.” Similarly, in June 2024, the SEC charged the CEO and founder of AI recruitment startup Joonko for misrepresenting the sophistication of its automation technology. The SEC emphasized that investors “considered the state of Joonko’s technology important in deciding whether to invest.”[20] While AI washing made up only a small piece of SEC enforcement in 2024, emerging financial technologies, including AI, are currently a component of the SEC’s examination priorities for 2025.[21]
Meanwhile, the FTC, in an enforcement sweep dubbed “Operation AI Comply” took action under the FTC Act against multiple companies using AI to engage in allegedly deceptive or unfair trade practices, such as promoting an AI tool used to create fake product reviews, providing an “AI Lawyer” service and selling products that claimed to use income-generating AI. The FTC is focused on combating AI systems “designed to deceive” and bogus claims of AI capabilities made to deceive consumers.
AI Governance Considerations
Over the next 24 months, more companies are expected to implement advanced, tailored AI solutions, in the hope of still greater benefits.[22] These opportunities to build a more competitive business should be a discussion topic for every board of directors and senior management team, and so too should the framework for evaluating and overseeing the attendant risks.
Risk Assessment Framework
For efficient yet measured governance, companies should consider implementing a risk assessment framework of delegation to vet low-risk AI tools and escalation to vet high-risk AI tools.
Risk “scorecards” can be used to internally standardize an initial risk assessment of proposed AI tools. Under this approach, the risk assessment team assigns a score to each category of risk (commercial risk, legal and regulatory risk, reputational risk, etc.) based on the likelihood of a liability-creating or otherwise damaging event and the potential impact of the event. Preparation of sample scorecards (i.e., for AI tools already vetted and deployed) may be a useful exercise for gaining perspective on the overall levels of risk represented by these scores.
This system of escalation affords boards and senior management the opportunity to make strategic decisions with respect to high-risk, high-reward AI use-cases while streamlining adoption of well-tested, low-risk AI tools. Outlined below are a few key considerations for boards when designing AI governance protocols.
AI Strategy: Risk Implications of Build versus Buy
The decision to build or buy generative AI solutions is primarily a commercial decision, but with meaningful risk implications. Only a small percentage of companies are building generative AI solutions fully in-house. Those that do may incur significant expense,[23] but they exercise more control over the technology, have the ability to customize it to their specific needs and can mitigate risks associated with outsourced AI infrastructure, such as data privacy.[24] Most companies have instead opted to buy or lease generative AI from third-party vendors or have partnered with vendors to build generative AI solutions, which can reduce development expense but increases third party risk exposure.[25]
Regardless of which strategy they adopt, boards and senior management should consider the benefits of creating a dedicated internal AI team or taskforce to assess the selection, implementation and risks of the chosen strategy. Responsibilities of such a team would include conducting due diligence on external AI tools,[26] fine-tuning AI models with company-specific data[27] and auditing existing AI models.[28] In particular, as more AI models are trained using synthetic (i.e., AI-generated) training data,[29] boards and management teams should be aware of the associated risks and appropriate safeguards.[30]
AI-Related Cybersecurity Risks
The FBI has warned about an increasing threat of cyber criminals using AI in cyberattacks, such as AI-driven phishing and AI-powered voice and image fraud.[31] Cyber criminals also target AI models themselves. Sophisticated cyberattacks on AI models, including data reconstruction attacks, create significant risk when the model is trained on highly sensitive data.[32] Some examples of highly sensitive data are health data, consumer data and personally identifiable information.[33]
Management teams, under board supervision, should have a protocol for scrutinizing the cybersecurity and debugging safeguards of every AI tool used in their companies, with input from internal cybersecurity, information technology and AI teams. With external AI tools, such as enterprise or open source AI, management should consider the single point of failure risk that, in the worst case, can lead to industry-wide crisis like the CrowdStrike outage.[34]
Accounting for AI Error
Because generative AI models are designed to be creative, some experts believe AI hallucination is not a solvable problem.[35] Yet, as the technology improves, there is a risk that employees might become over-reliant on AI and too trusting of its results.[36] There is also the potential black-box problem, where AI users are unable to understand how the AI makes decisions due to its complexity.[37]
Employees must be adequately trained to integrate AI into their work while also monitoring AI output for errors. In addition, boards of companies that manage sensitive or confidential data should ensure employees are aware of risks in submitting that data to AI products sold or leased by third parties.
Key Takeaways
- Novel AI copyright issues are being litigated, and courts will soon provide at least some answers to questions, such as the application of the fair use doctrine to various uses of AI.
- Though federal AI legislation is not expected soon, state lawmakers have been relatively proactive with respect to AI, addressing issues such as employment and consumer protection. Likewise, the EU has adopted its own comprehensive AI regulatory scheme with broad jurisdiction.
- Even without new AI-specific legislation, federal agencies have shown willingness to engage with AI-related issues and bring enforcement actions under existing law.
- With the variety of legal risks in mind, a risk assessment framework designed around a combination of delegation and escalation lets boards and management streamline the AI vetting process and appropriately shifts board-level focus to high-risk AI tools.
- Boards should consider the specific benefits and drawbacks of relying on third-party vendors versus in-house generative AI creation; each option offers different benefits and presents a different risk profile.
- It remains critical for companies to prioritize updating their cybersecurity infrastructure and risk frameworks for the rollout of generative AI.
- Corporate adoption of AI tools presents unique employee-level risks, the mitigation of which will require more than just vetting.
[1] Various federal government agencies issued a Joint Statement on Enforcement of Civil Rights, Fair Competition, Consumer Protection, and Equal Opportunity Laws in Automated Systems, available here. The Examination Division of the SEC named AI as a priority in its FY2025 Examination Priorities, available here. FSOC cohosted a conference on AI with the Brookings Institution, as described in its press release, available here.
[2] Our previous coverage of the EU AI Act can be found in our blog posts available here and here.
[3] See, e.g., Bloomberg Law, “AI-Washing Enforcement Crackdown Set to Survive Trump Rollbacks” (November 25, 2024), available here.
[4] 1:20-cv-00613 (D. Del. 2020).
[5] 3:23-cv-03417 (N.D. Cal. 2023).
[6] For additional discussion on earlier decisions in Kadrey, see our February 2024 blog post available here.
[7] 5:24-cv-03811 (N.D. Cal. 2024).
[8] For additional discussion of the Concord case, see our June 2024 blog post available here.
[9] 1:23-cv-11195 (S.D.N.Y. 2023).
[10] 3:23-cv-00201 (N.D. Cal. 2023).
[11] See U.S. Copyright Office, “Copyright and Artificial Intelligence Part 1: Digital Replicas” (July 2024), available here.
[12] Utah’s AI Policy Act can be found here.
[13] The Colorado AI Act can be found here.
[14] Those that make, or are a substantial factor in making, a “consequential decision.” Consequential decisions are those with a material legal or similarly significant effect on the provision or denial to any Colorado resident, or cost or terms of education, employment, financial/lending services, government services, healthcare, housing, insurance or legal services.
[15] The Generative AI Copyright Disclosure Act can be found here.
[16] See, for example the NIST Artificial Intelligence Risk Management Framework: Generative AI Profile that lays out 200+ suggested actions to mitigate the risk of generative AI, found here. Our previous discussion can found in our August 2024 blog post available here.
[17] AI systems are considered high-risk if they pose a “significant risk” to an individual’s health, safety, or fundamental rights, and, in particular, if: (i) they are intended to be used as a product or as a safety component of a product covered by EU harmonization legislation listed in Annex I (e.g., medical devices, industrial machinery, toys, aircraft, and cars) and the product is required to undergo a third-party conformity assessment under the above-mentioned legislation; or (ii) they are used in certain contexts listed in Annex III (e.g., AI systems used for education, employment, critical infrastructure, essential services, law enforcement, border control, and administration of justice). Obligations relate to risk management system, data governance, technical documentation, transparency, registration and record-keeping requirements and human oversight, as well as accuracy, robustness and cybersecurity.
[18] A GPAI model is defined as an: “AI model […] that displays significant generality and is capable of competently performing a wide range of distinct tasks regardless of the way the model is placed on the market and that can be integrated into a variety of downstream systems or applications”.
[19] For further information, please see the SEC’s March 2024 press release here.
[20] For further information, please see the SEC’s June 2024 press release here.
[21] The SEC’s 2025 Examination Priorities can be found here.
[22] See Microsoft, “IDC’s 2024 AI opportunity study: Top five AI trends to watch” (November 12, 2024), available here.
[23] See Time, “The Billion-Dollar Price Tag of Building AI” (June 3, 2024), available here.
[24] See, e.g., EY, “How organisations can choose between buying and building AI systems” (February 19, 2024), available here.
[25] KPMG surveyed 225 senior business leaders at companies with revenue greater than or equal to $1 billion in August 2024. Only 12% of companies are building generative AI in-house. 50% are buying or leasing generative AI from vendors, and 29% are pursuing a mix of building, buying, and partnering. See KPMG,
“GenAI Dramatically Shifting How Leaders Are Charting the Course for Their Organizations” (August 15, 2024), available here.
[26] For a risk assessment guide for AI vendors, see FS-ISAC, “Generative AI Vendor Risk Assessment Guide” (February 2024), available here, For a risk assessment guide for open source AI tools, see LeadDev, “Be careful with ‘open source’ AI” (August 20, 2024), available here.
[27] See, e.g., IBM, “What is fine-tuning?” (March 15, 2024), available here.
[28] For a general explanation of AI auditing, see Salesforce, “Are you ready for an AI audit?” (June 17, 2024), available here.
[29] See, e.g., IBM, “Examining synthetic data: The promise, risks and realities” (August 20, 2024), available here.
[30] Recent research has shown that indiscriminate use of online AI-generated text to train large-language models may cause irreversible defects in the resulting AI model, also known as model collapse. Shumailov et al., “AI models collapse when trained on recursively generated data” (July 24, 2024), available here.
[31] FBI, “FBI Warns of Increasing Threat of Cyber Criminals Utilizing Artificial Intelligence” (May 8, 2024), available here.
[32] For a more comprehensive overview of cybersecurity attacks on AI models, see Zohra El Mestari et al., “Preserving data privacy in machine learning systems” (February 2024), available here.
[33] Health information is protected by the HIPAA Privacy Rule. See U.S. Department of Health and Human Services, “The HIPAA Privacy Rule,” available here. Consumer privacy laws have been passed in 20 states. Bloomberg Law, “Twenty States Have Consumer Privacy Laws; More Likely to Come” (September 13, 2024), available here. For an overview of personally identifiable information, see, e.g., National Institute of Standards and Technology, “Guide to Protecting the Confidentiality of Personally Identifiable Information (PII)” (April 2010), available here.
[34] For a discussion on single point of failure risks generally, see Law.com, “CrowdStrike Glitch Highlights Risk of Single Point of Failure in Cybersecurity” (July 30, 2024), available here. For a discussion on the concentration of generative AI technologies, see MIT Technology Review, “Make no mistake—AI is owned by Big Tech” (December 5, 2023), available here.
[35] See Scientific American, “AI Chatbots Will Never Stop Hallucinating” (April 5, 2024), available here.
[36] See Stanford University, “Artificial Intelligence Index Report 2024,” Chapter 4, page 64 (May 2024), available here.
[37] For a discussion on AI’s black box problem, see World Economic Forum, “Building trust in AI means moving beyond black-box algorithms. Here’s why” (April 2, 2024), available here.